| Congratulations to Abhishek Singh Sambyal! Awarded Science and Engineering Research Board (SERB) Internation Travel Grant to attend MICCAI 2024, Morocco. |
| Congratulations to Abhishek Singh Sambyal! Awarded Medical Image Computing and Computer Assisted Intervention (MICCAI) 2024 RISE Registration Grant |
| Congratulations to Ranjana Roy Chowdhury! Awarded Society of Women Engineers (SWE) Scholarship 2024 - 2025 |
| Accepted to MICCAI 2024! LS+: Informed Label Smoothing for Improving Calibration in Medical Image Classification Abhishek Singh Sambyal, Usma Niyaz, Narayanan C. Krishnan and Deepti R. Bathula MICCAI 2024 abstract | bibtex | arXiv Deep Neural Networks (DNNs) exhibit exceptional performance in various tasks; however, their susceptibility to miscalibration poses challenges in healthcare applications, impacting reliability and trustworthiness. Label smoothing, which prefers soft targets based on uniform distribution over labels, is a widely used strategy to improvemodel calibration. We propose an improved strategy, Label Smoothing Plus (LS+), which uses class-specific prior that is estimated from val- idation set to account for current model calibration level. We evaluate the effectiveness of our approach by comparing it with state-of-the-art methods on three benchmark medical imaging datasets, using two different architectures and several performance and calibration metrics for the classification task. Experimental results show notable reduction in calibration error metrics with nominal improvement in performance compared to other approaches, suggesting that our proposed method provides more reliable prediction probabilities. Our code will be made available on GitHub after acceptance.
@inproceedings{Sambyal2024MICCAI,
title={LS+: Informed Label Smoothing for Improving Calibration in Medical Image Classification},
author={Sambyal, Abhishek Singh and Niyaz, Usma and Krishnan, Narayanan C. and Bathula, Deepti R.},
booktitle={MICCAI},
year={2024}} |
| Congratulations to Usma Niyaz! Awarded Science and Engineering Research Board (SERB) Travel Grant to attend ISBI 2024, Athens, Greece |
| Congratulations to Usma Niyaz! Awarded 2024 IEEE International Symposium on Biomedical Imaging (ISBI) Travel Grant |
| Best Student Paper Award! Mutually Exclusive Multi-Modal Approach for Parkinson’s Disease Classification Arunava Chaudhuri, Abhishek Singh Sambyal and Deepti R. Bathula Bioimaging 2024 abstract | bibtex | arXiv Parkinson's Disease (PD) is a progressive neurodegenerative disorder that affects the central nervous system and causes both motor and non-motor symptoms. While movement related symptoms are the most noticeable early signs, others like loss of smell can occur quite early and are easy to miss. This suggests that multi-modal assessment has significant potential in early diagnosis of PD. Multi-modal analysis allows for synergistic fusion of complementary information for improved prediction accuracy. However, acquiring all modalities for all subjects is not only expensive but also impractical in some cases. This work attempts to address the missing modality problem where the data is mutually exclusive. Specifically, we propose to leverage two distinct and unpaired datasets to improve the classification accuracy of PD. We propose a two-stage strategy that combines individual modality classifiers to train a multi-modality classifier using Siamese network with Triplet Loss. Furthermore, we use a Max-Voting strategy applied to Mix-and-Match pairing of the unlabelled test sample of one modality with both positive and negative samples from the other modality for test-time inference. We conducted experiments using gait sensor data (PhysioNet) and clinical data (PPMI). Our experimental results demonstrate the efficacy of the proposed approach compared to the state-of-the-art methods using single modality analysis.
@inproceedings{Chaudhuri2024MEMMPD,
title={Mutually Exclusive Multi-Modal Approach for Parkinson’s Disease Classification},
author={Chaudhuri, Arunava and Sambyal, Abhishek Singh and Bathula, Deepti R.},
booktitle={Bioimaging},
year={2024}} |
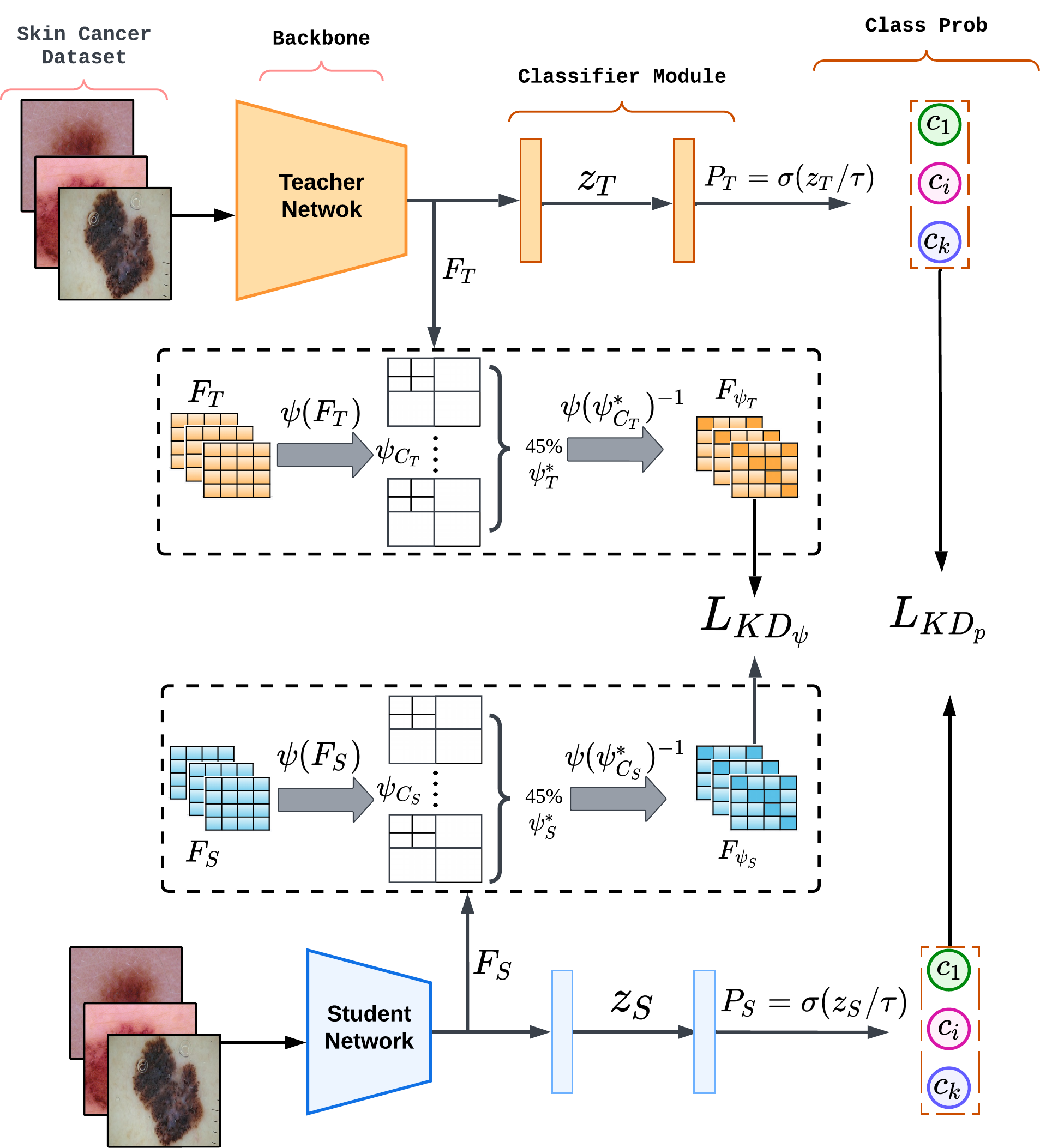 | Accepted to ISBI 2024! Wavelet-Based Feature Compression For Improved Knowledge Distillation Usma Niyaz, Abhishek Singh Sambyal and Deepti R. Bathula 21st IEEE International Symposium on Biomedical Imaging 2024 abstract | bibtex | arXiv Deep learning (DL) models can achieve state-of-the-art performance but at the cost of high computation and memory requirements. Due to their large capacity, DL models have the tendency to learn redundant features. In this work, we exploit this redundancy to improve model compression. Knowledge distillation (KD) aims to achieve model compression by transferring knowledge from a large, complex model to a simple, lightweight model. We propose an enhanced KD strategy that improves the efficiency of the distillation process by compressing the feature maps using Discrete Wavelet Transformation DWT, which helps capture crucial features from complex biomedical signals. Retaining and sharing only the most informative and discriminating features facilitates more effective feature mimicking. Extensive experiments using two benchmark datasets for Melanoma and Histopathology image classification tasks demonstrate the superiority of our proposed method over existing techniques. We further establish the generalizability and robustness of our method using two different teacher-student architectures and ablation studies.
@inproceedings{Niyaz2024ISBI,
title={Wavelet-Based Feature Compression For Improved Knowledge Distillation},
author={Niyaz, Usma and Sambyal, Abhishek Singh and Bathula, Deepti R.},
booktitle={ISBI},
year={2024}}
|